Industry 4.0 brings innovation to the manufacturing sector through the use of artificial intelligence (AI) and machine learning (ML) and real-time data collection and analysis. These processes are made possible by the Internet of Things (IoT) and cloud computing.
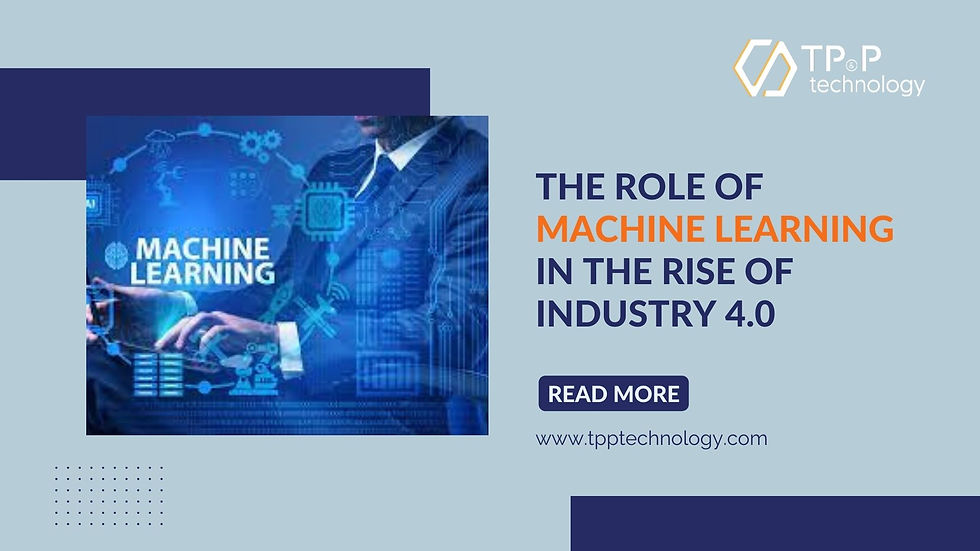
Smart factories use advanced sensors, embedded software and robotics to collect and analyze data to make better decisions. ML systems leverage information from manufacturing operations, enterprise resource planning (ERP), supply chain, customer relationship management (CRM), and other business systems to generate valuable insights. The entire process includes automation, enabling predictive maintenance and creating optimal process efficiency with greater responsiveness to customer needs.
In this article, we take a closer look at the role of machines in the development of Industry 4.0.
Intelligent quality control
Process-related data analysis combined with ML to identify errors and improve quality control. Machine vision technology provides information for ML defect detection. It automates large-scale product inspection with high accuracy and is highly effective in identifying complex defects that cannot be seen by the human eye.
ML tests non-contact manufactured products using IoT sensors. The algorithm searches the data stream for patterns that correspond to the damaged entities (on which the ML algorithm was previously trained). This process allows for quick identification of defective products and quick response by quality control specialists.
Better predictive maintenance
ML is very useful in predictive maintenance. It allows manufacturers to properly adjust, repair and maintain machines before costly damage occurs.
Predictive maintenance uses IoT sensors to collect data about the operating conditions and health of machines. Data collection can include readings for lubricants, energy loads, humidity, temperature, operating noise levels and more. The ML algorithm analyzes patterns in the collected data over time to reasonably predict when the machine will need maintenance. The system contains applicable standards for operating parameters to identify anomalies. ML dynamically adapts to new data in real-time and can detect and alert human staff to serious issues.
Improved supply chain
An improved supply chain is another example of machine learning use cases in production. A supply chain is now a non-deterministic workflow where the sequence must be adjusted and changed to optimize the process. Automation is needed to increase the effectiveness of supply chain management by increasing its visibility.
Artificial intelligence and machine learning for supply chain management automate repetitive tasks and streamline the process, forecast demand across product segments and geographies, adapt to volatility effects such as spikes in demand, and more. AI-based solutions are trained on data from material procurement, logistics and transportation systems to predict supply and demand. With all business needs in mind, this technology can improve purchasing, order fulfilment, inventory management, transportation logistics and delivery methods and times to the end user.
Site-note:
Need Help With Your Software Projects? Contact TP&P Technology - Leading Software Engineering Company in Vietnam Today
Comments